How AI is being used to predict natural disasters
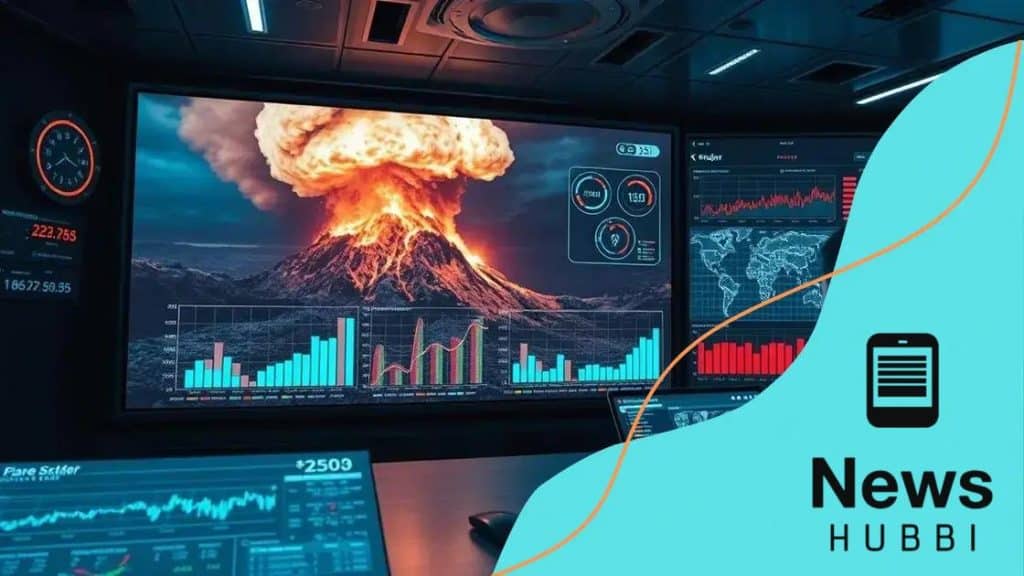
Anúncios
AI is being used to predict natural disasters by analyzing vast data sets to identify patterns, enabling faster and more accurate forecasting that enhances emergency response efforts.
How AI is being used to predict natural disasters is a crucial topic as climate patterns shift. Have you ever wondered how technology helps us anticipate such events and save lives? Let’s dive into it.
Anúncios
Understanding AI in disaster prediction
Understanding how AI operates in disaster prediction is essential for grasping its impact on safety and emergency response. At its core, AI processes vast amounts of data to identify patterns and forecasts.
The Role of Data in AI
Data serves as the backbone of AI applications. Various sources contribute to this pool of information:
- Satellite imagery helps track environmental changes.
- Social media feeds provide real-time updates from affected areas.
- Weather data predicts conditions leading to natural disasters.
By analyzing these data streams, AI systems can generate more accurate forecasts than traditional methods.
Anúncios
Machine Learning Techniques
Machine learning, a key component of AI, allows systems to improve over time. Algorithms learn from previous data, enhancing predictions for future events. For instance, when a system detects an increase in seismic activity, it can forecast earthquakes and send alerts to potentially affected areas.
This use of machine learning not only adds speed but also boosts precision in disaster prediction. As AI technologies evolve, their ability to analyze data swiftly will become more critical.
Moreover, AI’s integration with established warning systems can elevate public safety. Communities can benefit from timely alerts, leading to effective evacuations and preparedness strategies.
Understanding AI in disaster prediction is vital as it brings hope in minimizing loss of life and property during crises. By merging technology with real-time data, we are paving the way for a safer future.
Data sources for AI disaster forecasting
Data sources play a crucial role in AI’s ability to forecast disasters effectively. The foundation of reliable predictions lies in the quality and variety of data collected. These resources come from different domains, working together to enhance the capabilities of AI systems.
Types of Data Sources
AI uses multiple data types to paint a complete picture of potential disaster scenarios:
- Satellite imagery: This provides detailed views of geographical changes and weather patterns.
- Weather data: Accurate weather predictions help evaluate risk levels before disasters strike.
- Seismic sensors: These detect tremors and shifts in the earth’s crust, aiding in earthquake predictions.
- Social media information: Real-time updates from affected individuals can inform AI models.
By integrating these diverse data sources, AI creates a comprehensive approach to understanding and predicting disasters. This multi-faceted strategy is necessary for effective emergency response.
Importance of Real-Time Data
Real-time data is particularly vital in disaster forecasting. It allows AI to respond swiftly to developing situations. For example, during a hurricane, up-to-date weather reports enable the system to adjust predictions based on the latest information.
The integration of data sources also helps communities stay alert and be prepared for potential incidents. When people receive timely alerts, they can take necessary precautions to ensure their safety.
In summary, the continuous flow of diverse and real-time data enhances AI’s predictive accuracy, thus significantly improving disaster management efforts.
Machine learning techniques in action
Machine learning plays a vital role in enhancing AI’s ability to predict disasters accurately. By analyzing patterns in large data sets, machine learning enables systems to make predictions about potential disasters based on historical data.
Key Machine Learning Techniques
Several techniques stand out in the field of machine learning:
- Supervised learning: This technique uses labeled data to train AI models, helping them recognize patterns and make accurate predictions.
- Unsupervised learning: Without labeled data, this technique allows AI to identify hidden patterns and group similar data, providing insights into possible disaster scenarios.
- Reinforcement learning: This involves training an AI through trial and error, optimizing its responses based on feedback. In disaster prediction, it can improve decision-making over time.
These machine learning techniques are crucial as they allow AI to continuously improve its predictive capabilities. By learning from new data, AI can adjust its predictions and respond more effectively.
Practical Applications
In practice, machine learning models are being applied to various disaster scenarios. For instance, they help forecast hurricanes by analyzing weather conditions and historical patterns. When a system detects specific weather indicators, it can issue timely alerts, saving lives.
Moreover, predictive models are employed in wildfire management. By assessing vegetation moisture and weather data, AI helps determine the likelihood of wildfires, enabling rapid response efforts.
Machine learning techniques not only enhance accuracy but also speed up the response time during disasters. As these technologies advance, they hold the potential to revolutionize disaster predictions.
Benefits of AI for emergency management
The integration of AI in emergency management brings significant improvements to how disasters are handled. With AI, agencies can respond faster and more effectively, ultimately saving lives and resources.
Enhanced Decision-Making
One of the major benefits of AI is its ability to analyze data swiftly. By processing large amounts of information, AI helps emergency managers make informed decisions.
- Real-time analytics: AI provides instant insights from various data streams, aiding in timely responses.
- Predictive modeling: By forecasting potential disasters, AI enables better preparation and resource allocation.
- Strategic planning: AI models assist in simulating different disaster scenarios, helping to formulate effective response strategies.
This intelligent analysis allows for more precise actions during emergencies, which is critical in saving lives.
Improved Resource Allocation
AI also optimizes resource distribution during crises. Understanding the most affected areas allows for a better deployment of personnel and equipment. Emergency management can benefit from:
- Efficiency in logistics: AI streamlines supply chain operations to ensure timely delivery of necessities.
- Identifying hotspots: AI can identify where resources are needed most, maximizing their impact.
- Minimizing waste: Predictive capabilities ensure that resources are allocated appropriately, reducing unnecessary expenditure.
This efficient management is vital for effective response during crises. AI helps ensure that emergency services are available where they are most needed.
By enhancing decision-making and optimizing resource allocation, the benefits of AI for emergency management are clear. These advancements lead to better outcomes during disasters, ultimately improving community safety.
Challenges in AI disaster prediction
While AI significantly enhances disaster prediction, there are important challenges that need to be addressed. Understanding these hurdles is crucial for improving current technologies.
Data Quality Issues
The success of AI relies heavily on the quality of data provided. If the data is inaccurate or incomplete, predictions may fail. Incomplete datasets can lead to incorrect forecasting, which puts lives at risk.
- Inconsistent data collection methods can create gaps.
- Data from different sources may not align well.
- Outdated information can mislead predictions.
Ensuring high-quality and timely data is essential for reliable predictions.
Algorithm Limitations
Algorithms used in AI also come with their own limitations. Most models require constant updating and training. Without regular improvements, they may not adapt well to new situations.
Additionally, black box algorithms can make it difficult to understand how decisions are made. This lack of transparency can hinder trust among emergency management personnel.
Some machine learning models can also struggle with rare events, making them less effective for predicting infrequent but devastating disasters.
Ethical Concerns
Ethical challenges pose another significant barrier. The use of personal data, especially from social media, raises privacy concerns. People may not realize their information is being used in AI models.
Moreover, relying too much on AI could lead to overconfidence. If decisions are solely based on predictions, humans may neglect to apply common sense and judgment during emergencies.
Addressing these challenges is essential for maximizing the benefits of AI in disaster prediction. Overcoming data quality issues, algorithm limitations, and ethical concerns will pave the way for more effective AI systems.
In summary, AI is reshaping how we predict and manage natural disasters. It offers significant benefits like improved decision-making and efficient resource allocation. However, challenges such as data quality, algorithm limitations, and ethical concerns must be addressed. By overcoming these obstacles, we can leverage AI’s full potential to enhance public safety and save lives during emergencies. As technology continues to evolve, the collaboration between AI and human expertise will be vital in creating a safer and more prepared future.
\n\n
\n
FAQ – Frequently Asked Questions about AI in Disaster Prediction
How does AI improve disaster prediction?
AI enhances disaster prediction by analyzing large data sets to identify patterns, enabling more accurate forecasts and timely alerts.
What are some challenges faced by AI in disaster management?
Key challenges include data quality issues, algorithm limitations, and ethical concerns regarding data privacy.
How can data quality impact disaster predictions?
Poor data quality can lead to inaccurate forecasts, potentially risking lives during emergencies by providing false security.
Can AI help in resource allocation during emergencies?
Yes, AI optimizes resource allocation by analyzing data to determine where resources are needed most during disasters.